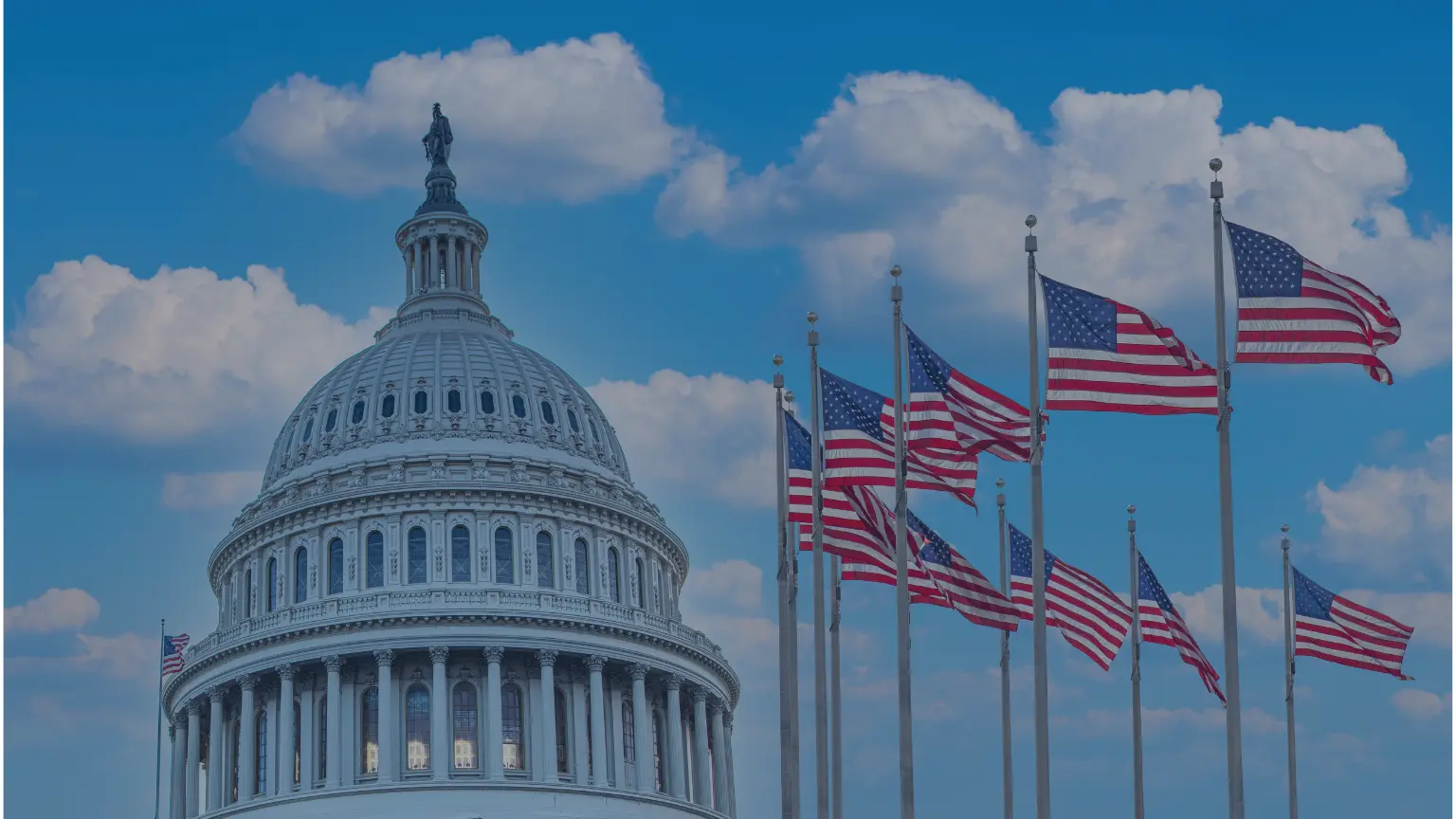
What is Data Governance: The Ultimate guide for small businesses
Data governance controls data flow to stakeholders who need it and is now vital to every company’s data strategy.
As the amount of data in your organization increases, all business processes profit from being data-driven because data helps people make better, more educated decisions.
Naturally, the growth of data also brings with it additional challenges. Your business will encounter more data problems as more data assets are collected.
Organizations often lose control over the data lifecycle when more data items pour in from dozens or hundreds of sources.
If you can even fulfill the expectations of end consumers, scaling to do so becomes more challenging.
Effective data governance allows tracking when and from where incorrect data enters your systems and who uses it.
The absence of data governance results in poor data quality and decreases the confidence of your stakeholders in the data.
Data concerns also raise the possibility of failing to adhere to business and governmental regulations like the Global Data Protection Regulation (GDPR).
Additionally, as data environments get more complex, costs rise due to maintaining them.
Because of this, effective data governance methods that ensure data privacy and compliance are now crucial for companies to thrive.
In this article, we will cover it all for you: what is data governance framework, its benefits for data-driven organizations, data governance vs. data management, core elements of data governance framework, examples, templates and how to create a data governance framework for your organization.
What is a Data Governance Framework?
To help you understand data governance frameworks, let’s start by defining what data governance means.
Data Governance
Data governance includes the strategies and procedures necessary to effectively handle enterprise data, utilizing it for informed business decisions, ultimately making a lasting impact on business’ bottom line.
In essence, data governance involves the management of all the assets related to an organization’s information—not just data but also documents, applications, networks, configurations, and metadata.
Data Governance Framework
A data governance framework, similar to a structured set of guidelines and procedures, outlines data management in a firm.
This covers every aspect, from data collection and storage to data usage.
A business can transform its data into a helpful resource by adhering to this framework correctly.
Therefore, the company’s aims and objectives can be attained or surpassed using this data.
Every business is led by a set of business drivers, which are crucial elements or procedures for the company’s ongoing success.
What data needs to be carefully controlled and to what extent your data governance strategy depends on the specific business drivers of your firm.
For instance, a healthcare organization may need to keep patient information private.
This is important for following laws and industry standards. The organization must manage the data securely as it moves through the company.
The patient’s healthcare providers must also have easy access to the patient’s data.
As the foundation of its data governance framework, the supplier builds its data governance approach on these needs.
The tasks and responsibilities of a well-designed data governance framework include strategic, tactical, and operational aspects.
It ensures that data is reliable, well-documented, and easily accessible within your business.
It also ensures that the data is secure, compliant, and private.
Benefits of Data Governance Framework
Data is the costliest asset thanks to its ability to retain valuable information, which when analyzed, helps businesses make informed decisions and build impeccable brands in the modern digital landscape.
Assuring the security of stored data is a significant duty that goes along with that ability.
Even though laws and regulations have increased compliance and overall data security, breaches that leak customers’ personal information continue to make headlines daily.
Additionally, it prompted businesses to take additional careful measures to protect their data.
With the implementation of data governance practices, businesses can improve security and gain other significant economic advantages.
The following are the main benefits of sound data governance:
- Improved decision support: Ensure that data is reliable and consistent across the company to foster trust and generate better insights to support decision-making.
- Risk reduction: Encourages regulatory compliance, lowering risks that may otherwise jeopardize an organization’s lifespan and data security.
- Better scalability: This allows for smooth expansion despite considerable increases in data volume.
- Increased productivity: A company’s IT department will be more adaptable and scalable by establishing transparent procedures and guidelines for data management and strategy.
- Reduced expenses measure: Centralizes data control to avoid the costs of segregated or fragmented data management.
- Strengthens collaboration: Collaboration is supported by providing accurate, consistent data throughout the company and encouraging a collaborative culture.
Data Governance vs. Data Management
Even though the phrases data management and data governance are sometimes used interchangeably, they have different meanings.
Data governance establishes guidelines for properly using, processing and storing information related to an organization’s broader business data strategy.
The organization’s data governance vision and framework are created by executives, stakeholders, and data trustees.
Data management refers to the tactics employed to carry out the data governance strategy, ensuring that policies throughout its entire lifecycle manage data.
Data security and quality, master data management, and database operations are also included in data management.
The responsibilities of data custodians and stewards are to implement and maintain technology and uphold the rules and regulations established in the data governance framework.
Both disciplines collaborate to maintain corporate data as a valuable business asset.
This is particularly significant as companies move their master data to the cloud, where effective information governance and data management are essential for a smooth transition.
Core Elements of a Data Governance Framework
A harmonious alignment of people, processes, and technology is imperative for a successful data governance program.
Establishing an appropriate framework to carry out the program effectively is equally vital, and this requires close cooperation among committed IT experts, business leaders, and stakeholders involved in data management.
Outlined below are the core elements to incorporate into your data governance framework:
- Data Strategy
A crucial first step in establishing a data governance framework is developing an intensive data strategy.
An organization’s broad operational rules and requirements about data are included in a data strategy, which frequently reflects the current organizational culture.
For instance, it includes deciding whether a liberal or restricted approach will be used, and the level of trust extended to internal users.
It also involves deciding on the data collection size, such as whether focused or comprehensive attempts will be made.
- Data Processes
Every data governance framework must have crucial processes in place for data management.
Standard practices cover sharing data, testing and monitoring data quality, recording data issues, etc.
- Data Policy
For enterprise data, a framework also creates a data governance policy.
Internal and external data sharing, regulatory compliance, data storage needs, data quality, etc., are a few examples of data policies.
- Data Standards
Data standards define the overall approach for assuring adherence to the data policy.
Data modeling standards, naming and abbreviation standards, metadata management, etc., are a few examples of data standards.
A marketing taxonomy, the standard design for producing marketing data (campaigns, assets, tags), is one of the most prevalent use cases for data standards.
- Data Security
Data security is the term used to describe protecting business data throughout its lifecycle.
The framework should include instructions on protecting data against theft, illegal access, cyberattacks, and other threats.
A precise action plan for dealing with potential risks should also be established.
- Data Quality
A data set’s ability to fulfill its business purpose depends on the data quality, so maintaining good data quality should be a top priority when you create your data governance framework.
An intelligent way to start is by establishing regulations that forbid manual entry, demand open standards, and limit data access.
Because poor data quality can ruin the success of campaigns, this can be the most essential part of any data governance framework.
- Collaboration & Communication
Data governance is a strategic effort that calls for company-wide work.
Therefore, it is crucial to have a plan for sharing the actions and successes of the data governance team.
Clearly describe objectives, aims, and tools to encourage passionate support and widespread adoption of a data governance framework.
The internal culture of your business about data sharing, communication, and cooperation may make or break data-driven efforts, just like the quality of your data.
- Data Literacy
For data governance to be successful, a considerable cultural shift may occasionally be required.
This can be achieved by encouraging data literacy or educating people.
Part of that education process includes prioritizing data democratization or ensuring all users can access, comprehend, and use data.
Better-informed users make decisions that utilize the potential of enterprise data.
- Key performance indicators (KPIs)
Establishing KPIs is essential to gauge the business impact of data governance projects, which demand a coordinated commitment in resources (people and money).
KPIs should also be quantifiable and tracked to give stakeholders a precise return on investment (ROI).
- Technology
The success of an organization’s data policy depends on the choice of data governance technologies for your specific needs.
Be sure to specify your requirements because standalone solutions and integrated data platforms offer many features.
Most data governance software platforms provide fundamental features like automating workflows, developing a dictionary of business terms, etc.
Many, like PrivacyPillar’s data privacy solutions, also provide a range of data capabilities, such as data management, integration, cleansing, and discovery.
Data Governance Framework Examples- Traditional Approach
Top-down and bottom-up are traditional approaches to formulating a data governance framework. These two approaches come from different ideas.
To improve data quality, one gives priority to the control of the data.
The other gives quick access to data as a top priority to maximize end users’ data access across business units.
- Top-down method
The centralized method of data governance looks like this.
It is supported by a small group of data experts who use established best practices and well-defined methodology.
Data modeling and control are therefore given priority.
The rest of the organization gets access to the data for analytics much later.
This method, meanwhile, produces a significant scaling issue.
This framework distinguishes between data consumers (usually business units) and data suppliers (often IT).
The data providers are the only people with control over the data.
This was less of a problem in the past because there was less data that needed to be managed and fewer teams that required access to it.
However, these days, these small teams of data producers are currently unable to meet the needs of data users.
The availability of clean, comprehensive, and unaffected data to anyone who wants it at any time has become a corporate need.
Simply put, business users have too many requests for these teams to continue acting as gatekeepers.
- Bottom-Up method
In contrast, the bottom-up approach to data management enables far greater agility.
Unlike the top-down approach, which begins with data governance and modeling, the bottom-up approach starts with raw data.
Structures can be built on top of the raw data (a process known as “schema on read”), and data quality controls, security rules, and policies can be implemented after the raw data has been ingested.
This framework, which gained popularity with the introduction of big data, is more scalable.
However, it raises an entirely new set of data concerns.
It is more difficult to establish control since data governance isn’t enforced until later in the process and because anyone can enter data.
Additionally, as we have already stated, a lack of data governance can increase regulatory risk, lose stakeholder confidence in the organization’s data, and increase the cost of data management for a disorganized, expansive collection of data assets.
We require a modern approach to data governance framework that strikes a balance between access and control.
Early in the process, we must establish control while allowing users and subject-matter experts to become data owners and curators.
Collaborative Data Governance Framework template – a modern approach
A collaborative data governance framework’s primary goal is to balance top-down and bottom-up approaches.
The success of this framework is based on teamwork with data; otherwise, the amount of labor required to validate the reliability of the data will be burdensome.
The collaborative framework is scalable, enabling more individuals in an organization to introduce more data sources.
Clear guidelines for collaborative content curation must be developed to keep this scalability.
This may entail choosing data stewards who are subject matter experts in each business unit to assist in maintaining excellent data quality for the datasets they are most experienced with.
These guidelines for data curation enable anyone to collaborate as long as they adhere to the rules.
This assures scalability while maintaining a certain level of content trust.
Businesses can involve every department, from IT to subject matter experts to decision-makers, in transforming unstructured data into reliable business data for sharing and use.
Undoubtedly, some business operations depend on strictly regulated data components that call for special attention.
For instance, data like consumer credit card information or risk data aggregation in financial services might not fit this approach best.
In these situations, the collaborative framework can supplement a more controlled top-down approach rather than take its place.
The organization’s data governance team should determine which data governance approach is appropriate in these circumstances.
How to create a data governance framework
Even though every business is unique, you shouldn’t have to reinvent the wheel to set up your framework.
Here are some best practices for data governance to help you instead.
- Think big but start small.
Finding a strategy to satisfy business goals and promote adoption among data consumers takes time, and effective data governance implementation must take time.
Starting with a quick win, such as improving the data quality in a few crucial business operations, will show the need to put corporate governance into practice.
- Identify champions
Gaining support requires educating stakeholders about the business advantages of data governance.
Technical components should be translated into applicable commercial terms to find supporters among important business areas and ensure the program’s success.
- Determine the roles and responsibilities.
Determining deliverables and ownership levels within the company lays the groundwork for what is frequently a major cultural and procedural transformation for an organization.
The standard responsibilities that should be established early on are listed below.
- Executive sponsor: C-level sponsor(s) who know the program’s objectives and ensure that it receives adequate resources.
- Data governance council: The data governance council directs the program’s strategy, sets priorities for projects and initiatives, and establishes data definitions, policies, and standards.
- Data governance board: The board is responsible for creating strategic procedures and guidelines to use data as a business resource.
- Data stewards: People who implement data governance practices to maintain the quality of data components and add order and value to unstructured data.
- Data owners: People in charge of the data for business operations.
- Data consumers: People who enter and use data to carry out their given tasks.
- Establish a business case.
For a data governance program to be successful at generating benefits throughout the entire organization, complete commitment and a significant investment of resources and time is required.
A strong business case might make persuading stakeholders to embrace the challenge easier.
Start by determining the fundamental data components and their underlying business functions.
The costs of integrating, administering, and validating those elements using the current manual processes are then described.
To highlight the benefit of implementing a data governance approach, emphasize the possible commercial consequences of manual process failures.
- Use metrics to measure progress.
The effectiveness of data governance programs may be proved using the insights obtained through constantly tracking and collecting metrics.
Metrics also show how well procedures and policies work and highlight improvement areas.
- Reward participation to encourage it.
Regular reports on organizational successes can help strengthen the essential cultural and procedural adjustments for long-term success; rewards are always helpful.
Consider implementing an incentive-based reward system to promote adoption and get the most out of a data governance program.
Conclusion: Unlocking the full potential of a data governance framework
Business units must see the data governance team as a friend and ally in their operational procedures across the enterprise.
After all, data security and sensitive data management are only a tiny part of governance.
Thanks to data governance policies, business users can access the required data when needed.
In the end, data governance serves as a tool to improve decision-making.
A culture that supports and encourages data governance can be developed using change management.
By convincing your business units that data governance practices, when properly implemented, don’t slow down business operations or restrict access to necessary data, you resolve worries about new data governance regulations.
Instead, these regulations should enable self-service delivery of reliable data to the appropriate audience in the proper format at the right time, all while protecting data privacy and regulatory compliance.
Informing data end users of the advantages data governance brings to their business operations will help them appreciate it.
For instance, automating data privacy regulations could enable data consumers to concentrate on data analysis and decision-making rather than worrying about how well their operations safeguard sensitive data.